Artificial Intelligence (AI) has become a big part of our daily lives. It helps suggest movies you might like, writes essays, or handles tasks at work. But there’s one question that makes people curious—can AI really think?
Thinking means understanding, solving problems, and making decisions with awareness. This is how humans adapt and figure things out. AI, however, doesn’t work the same way. It uses data and follows patterns and rules it has learned. Even though it might look like AI is thinking, it’s actually just copying how humans think without really understanding.
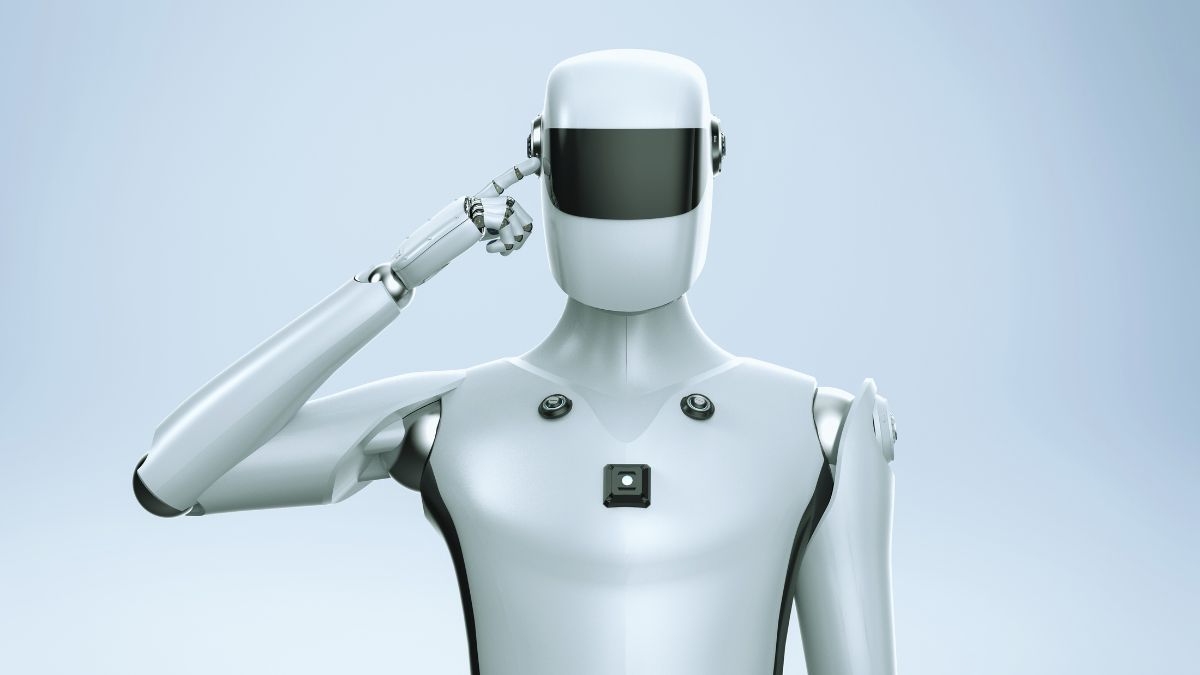
What Does It Mean for AI to Think?
For humans, thinking is a conscious process. It involves understanding situations, analyzing information, and making decisions based on experiences or logic. For example, if you’re solving a puzzle, you might take a moment to analyze the pieces, try different approaches, and come up with a solution. This process requires reasoning and understanding.
AI doesn’t have the ability to think in the same way. Instead, it processes information using algorithms and patterns learned from its training data. This process is known as probabilistic pattern matching. Essentially, AI looks for patterns in its training data to predict the best possible response to a given input.
For example, if you ask an AI, “What is 2+2?” it will answer “4” because that’s the most common response in its training data. However, this doesn’t mean the AI understands what numbers are or how addition works. It simply predicts the correct answer based on the patterns it has learned.
Why AI Struggles with Reasoning
Artificial Intelligence (AI) has made significant strides over the years, but its ability to reason like humans remains limited. Although AI can process vast amounts of information and perform tasks at incredible speed, it still faces challenges when it comes to logical reasoning and understanding complex concepts. Below are three main reasons why AI struggles with reasoning.
1. Dependence on Training Data
One of the primary reasons AI struggles with reasoning is its complete dependence on training data. AI systems learn from data, which means that everything an AI knows is derived from what it has been trained on. If the data is wrong, biased, or incomplete, the AI can take those same issues, leading to incorrect conclusions or faulty reasoning.
Imagine training an AI on a dataset that predominantly features math problems where extra details often signal the need for subtraction. The AI might learn to associate certain types of language, like “decrease” or “less,” with subtraction, even when it’s not necessary. So, if a new math problem appears that doesn’t require subtraction, but contains similar phrasing, the AI might incorrectly apply subtraction because it learned this pattern during training.
Moreover, the quality and diversity of the data also affect the AI’s reasoning. If the training data doesn’t cover a wide range of examples or contexts, the AI may struggle to generalize to new, unfamiliar situations. For example, if an AI is trained on images of dogs and cats, it might not recognize a rabbit or a horse because it hasn’t encountered these animals in the training set. This highlights the importance of using comprehensive and unbiased data to improve the reasoning capabilities of AI.
2. Token Bias and Input Sensitivity
AI processes information in chunks known as tokens (small units of text or data that represent words, phrases, or even individual characters). These tokens help AI understand language by breaking it down into digestible parts. However, this process also introduces challenges when it comes to interpreting complex inputs. Even a minor change in how a question or input is phrased can dramatically alter the AI’s response, making it highly sensitive to wording and structure.
For example, suppose you ask the AI, “What is 10 apples plus 5 oranges?” The inclusion of the word “oranges” may confuse the AI because it may not know how to handle the information about different types of fruits within the same problem. The AI might focus too much on the word “oranges,” misinterpreting the question, and providing an irrelevant or incorrect response. This is called token bias, where the AI focuses on certain tokens in a way that alters the overall meaning or logic of the question.
3. Lack of True Understanding
Another major limitation of AI when it comes to reasoning is its lack of true understanding. AI doesn’t “know” things the way humans do. It doesn’t have consciousness or self-awareness, and it doesn’t comprehend concepts like “right” or “wrong.” Instead, it relies on algorithms and patterns to simulate reasoning by predicting likely answers based on the data it has been trained on. This lack of true understanding means that AI can’t make judgments or consider context in the way a human would.
For example, when asked to solve a riddle, an AI might attempt to find an answer by looking for patterns or similar riddles in its training data. However, riddles often contain deeper meanings or rely on abstract thinking that AI struggles to grasp. Take the classic riddle, “What has keys but can’t open locks?” AI might answer “keyboard” based on pattern recognition, but it might miss the broader idea that the riddle plays on the double meaning of the word “keys.” The AI doesn’t “understand” that riddles often rely on humor or creative thinking, it just predicts an answer based on its data.
How AI Simulates Thought
AI often gives answers that seem intelligent, but this is more like a highly advanced version of autocomplete.
Think about how your phone suggests the next word while you’re typing. For example, if you type “I am going to,” your phone might suggest “work” or “school.” It makes these suggestions based on patterns in your previous messages or common phrases people use.
AI works similarly but on a much larger scale. When you ask a question, the AI doesn’t truly understand it. Instead, it looks at patterns in its training data and predicts the next best word, sentence, or paragraph. For example, if you ask an AI , What is the capital of France? it will answer Paris because that’s the most common answer in its training data. However, if the question is confusing, the AI might give a wrong answer because it’s not reasoning, it’s just predicting the answers based on patterns.
Recent Advances in AI Reasoning
Artificial Intelligence (AI) has made significant advancement in recent years, especially in the area of reasoning. While AI systems are still far from being able to think like humans, several advancements have improved their ability to solve problems and provide more accurate answers. Two key developments Chain of Thought Prompting and Inference Time Compute are playing a major role in improving AI’s reasoning capabilities.
1. Chain of Thought Prompting
Chain of thought prompting is a method that encourages AI to break down complex problems into smaller, more manageable steps. This technique mimics the way humans approach problem-solving by thinking things through logically and step by step. The idea behind chain of thought prompting is simple: instead of giving an answer right away, the AI is prompted to reason through the problem and explain its thought process.
Take a simple math problem, for example: “What is 5+3+2?” Without a chain of thought prompting, an AI might simply give the answer, “10.” However, with this method, the AI is instructed to first calculate 5+3, which equals 8, then add 2, which gives the correct final answer of 10. By breaking the problem into smaller steps, AI is less likely to make errors and can offer a more transparent process for users to follow.
2. Inference Time Compute
Inference time compute is another promising development in AI reasoning. Unlike traditional models that quickly generate answers based on pre-trained data, inference time compute allows AI to spend more time “thinking” before responding. This process gives the AI the ability to analyze a question or task more thoroughly, considering multiple factors and reasoning through the problem to arrive at the most accurate answer.
Imagine you ask an AI to summarize a research paper on climate change. With inference time compute, the AI won’t just give a quick answer. It will take a few extra moments to read through the entire paper, understand the key concepts, and logically organize the information before presenting a summary. This extra processing time allows the AI to consider more context and details, improving the accuracy of the response.
Related:
9 ChatGPT Prompts To Hack Customer Psychology And Dominate Your Niche
Can AI Truly Think?
While AI is no doubt powerful, it cannot truly think in the way humans do. Thinking, as we understand it, involves awareness, reasoning, and the ability to reflect on one’s own thoughts. Human thinking is deeply tied to consciousness—our emotions, experiences, and personal understanding of the world. AI, however, lacks these qualities. It doesn’t have emotions or a sense of self-awareness, and it doesn’t comprehend the information it processes.
The Nature of AI’s “Thinking”
AI’s “thinking” is more accurately described as pattern recognition. When you ask an AI a question or give it a task, it uses complex algorithms to analyze input and search for patterns in its training data. For example, if you ask an AI to suggest a recipe, it doesn’t “know” what ingredients taste good together. Instead, it searches through millions of recipe examples to find common combinations and then predicts the best answer based on those patterns.
Similarly, if you ask an AI for a weather forecast, it doesn’t have an understanding of what “rain” feels like or what it means to be cold. It simply pulls data from weather patterns and generates a forecast based on past information. In this way, AI mimics thinking by processing data in a way that seems intelligent, but it doesn’t truly understand the concepts it deals with.
AI and Its Limitations
The limitations of AI’s reasoning come from its lack of genuine understanding. For instance, AI may provide correct answers based on patterns but fail to adapt when it encounters unfamiliar or unusual situations. For example, if you ask an AI, “What’s the meaning of life?” it might provide a philosophical quote, but it doesn’t truly grasp the deeper implications of the question. It can’t reflect on its own existence or draw from personal experience, which are key aspects of human thinking.
Even more practical applications can highlight these limitations. In medical diagnostics, AI might correctly identify symptoms based on past data, but it doesn’t have the intuition or judgment that human doctors develop through years of experience and patient interaction. AI may not be able to consider the broader context of a patient’s health or lifestyle, which can be crucial in making accurate diagnoses.
AI as a Tool, Not a Thinker
Despite its inability to truly think, AI is an incredibly valuable tool. It can perform tasks quickly and accurately, assist with decision-making, and process large amounts of data that would take humans much longer to analyze. However, understanding AI’s limitations is crucial to using it effectively. While it may seem intelligent, it is still a machine following a set of instructions rather than an independent thinker. By recognizing this distinction, we can make the most of AI’s capabilities while avoiding unrealistic expectations.
The Future of AI Reasoning
AI reasoning is evolving rapidly, and researchers are hopeful that future models will exhibit even more sophisticated reasoning capabilities. While AI is still far from thinking like humans, there are exciting developments on the horizon that could help AI better understand and solve complex problems.
1. Improving Training Data
One of the biggest challenges in AI reasoning is ensuring the training data is high-quality and comprehensive. If an AI model is trained on incomplete or biased data, its reasoning can be flawed. For example, an AI trained only on images of dogs and cats might struggle to identify other animals, such as rabbits or birds. By improving the quality and diversity of training data, researchers aim to create AI systems that are more capable of understanding and responding to a wider range of problems.
Furthermore, by providing AI systems with data that includes logical reasoning examples, researchers can help them better simulate human-like thought processes. This would enable AI to not only recall information but also reason through situations with greater accuracy.
2. Enhancing Algorithms
Researchers are continuously developing new algorithms that allow AI to reason more effectively. For instance, the use of more advanced neural networks and machine learning techniques can help AI systems recognize patterns in data more accurately. These advancements could lead to better decision-making, especially in fields like healthcare, finance, and law.
One promising approach is meta-learning, where AI learns how to learn. This allows AI systems to adapt more quickly to new situations and improve their reasoning over time. Rather than being limited by the data they are trained on, these systems can apply learned strategies to solve novel problems and enhance their reasoning abilities.
3. Collaborative AI and Human Interaction
While AI reasoning is improving, it’s essential to remember that AI is a tool designed to complement human intelligence, not replace it. In the future, we’re likely to see more collaborative AI, where humans and AI work together to solve complex problems. AI can handle data analysis, pattern recognition, and other tasks, while humans bring creativity, empathy, and nuanced understanding to the table.
This synergy between human and AI reasoning could revolutionize fields like medicine, engineering, and science. For example, AI could process vast amounts of medical data, while doctors use their expertise and judgment to make final decisions. Together, they can arrive at solutions that neither could achieve alone.
Conclusion
AI is not capable of true thought, but it has the ability to simulate intelligent behavior through pattern recognition and data analysis. While it struggles with reasoning and lacks consciousness, advancements like chain of thought prompting and inference time computation are improving its problem-solving abilities.
As we look toward the future, AI’s reasoning capabilities will continue to improve. However, it’s important to remember that AI is a powerful tool designed to assist humans, not replace them. By understanding its strengths and limitations, we can use AI more effectively to enhance our lives and work.